A data frame is an R object that store data in two dimensions represented by columns and rows. The columns are the different variables of the dataframe and the rows are the observations of each variable. Each row of the dataframe represent a unique set of observations. This object is a useful data structure to store data with different types in. The Data Import cheatsheet reminds you how to read in flat files with work with the results as tibbles, and reshape messy data with tidyr. Use tidyr to reshape your tables into tidy data, the data format that works the most seamlessly with R and the tidyverse. Updated January 17.
Pandas can be used as the most important Python package for Data Science. It helps to provide a lot of functions that deal with the data in easier way. It's fast, flexible, and expressive data structures are designed to make real-world data analysis.
Dask collections (continued) custom computations for custom code and complex algorithms advanced dask delayed lazy parallelism for custom code. Dask Cheat Sheet ¶ The 300KB pdf Dask cheat sheet is a single page summary about using Dask. It is commonly distributed at conferences and trade shows. This PySpark SQL cheat sheet covers the basics of working with the Apache Spark DataFrames in Python: from initializing the SparkSession to creating DataFrames, inspecting the data, handling duplicate values, querying, adding, updating or removing columns, grouping, filtering or sorting data.
Pandas Cheat Sheet is a quick guide through the basics of Pandas that you will need to get started on wrangling your data with Python. If you want to begin your data science journey with Pandas, you can use it as a handy reference to deal with the data easily.
This cheat sheet will guide through the basics of the Pandas library from the data structure to I/O, selection, sorting and ranking, etc. Boss tone studio version 2 mac download.
Key and Imports
We use following shorthand in the cheat sheet:
- df: Refers to any Pandas Dataframe object.
- s: Refers to any Pandas Series object. You can use the following imports to get started:
Pandas Dataframe Plot Cheat Sheet
Importing Data
- pd.read_csv(filename) : It read the data from CSV file.
- pd.read_table(filename) : It is used to read the data from delimited text file.
- pd.read_excel(filename) : It read the data from an Excel file.
- pd.read_sql(query,connection _object) : It read the data from a SQL table/database.
- pd.read_json(json _string) : It read the data from a JSON formatted string, URL or file.
- pd.read_html(url) : It parses an html URL, string or the file and extract the tables to a list of dataframes.
- pd.read_clipboard() : It takes the contents of clipboard and passes it to the read_table() function.
- pd.DataFrame(dict) : From the dict, keys for the columns names, values for the data as lists.

Exporting data
- df.to_csv(filename): It writes to a CSV file.
- df.to_excel(filename): It writes to an Excel file.
- df.to_sql(table_name, connection_object): It writes to a SQL table.
- df.to_json(filename) : It write to a file in JSON format.
Create Test objects
It is useful for testing the code segments.
- pd.DataFrame(np.random.rand(7,18)): Refers to 18 columns and 7 rows of random floats.
- pd.Series(my_list): It creates a Series from an iterable my_list.
- df.index= pd.date_range('1940/1/20', periods=df.shape[0]): It adds the date index.
Viewing/Inspecting Data
- df.head(n): It returns first n rows of the DataFrame.
- df.tail(n): It returns last n rows of the DataFrame.
- df.shape: It returns number of rows and columns.
- df.info(): It returns index, Datatype, and memory information.
- s.value_counts(dropna=False): It views unique values and counts.
- df.apply(pd.Series.value_counts): It refers to the unique values and counts for all the columns.
Selection
- df[col1]: It returns column with the label col as Series.
- df[[col1, col2]]: It returns columns as a new DataFrame.
- s.iloc[0]: It select by the position.
- s.loc['index_one']: It select by the index.
- df.iloc[0,:]: It returns first row.
- df.iloc[0,0]: It returns the first element of first column.
Data cleaning
- df.columns = ['a','b','c']: It rename the columns.
- pd.isnull(): It checks for the null values and returns the Boolean array.
- pd.notnull(): It is opposite of pd.isnull().
- df.dropna(): It drops all the rows that contain the null values.
- df.dropna(axis= 1): It drops all the columns that contain null values.
- df.dropna(axis=1,thresh=n): It drops all the rows that have less than n non null values.
- df.fillna(x): It replaces all null values with x.
- s.fillna(s.mean()): It replaces all the null values with the mean(the mean can be replaced with almost any function from the statistics module).
- s.astype(float): It converts the datatype of series to float.
- s.replace(1, 'one'): It replaces all the values equal to 1 with 'one'.
- s.replace([1,3],[ 'one', 'three']):It replaces all 1 with 'one' and 3 with 'three'.
- df.rename(columns=lambda x: x+1):It rename mass of the columns.
- df.rename(columns={'old_name': 'new_ name'}): It consist selective renaming.
- df.set_index('column_one'): Used for changing the index.
- df.rename(index=lambda x: x+1): It rename mass of the index.
Filter, Sort, and Groupby
- df[df[col] > 0.5]: Returns the rows where column col is greater than 0.5
- df[(df[col] > 0.5) & (df[col] < 0.7)] : Returns the rows where 0.7 > col > 0.5
- df.sort_values(col1) :It sorts the values by col1 in ascending order.
- df.sort_values(col2,ascending=False) :It sorts the values by col2 in descending order.
- df.sort_values([col1,col2],ascending=[True,False]) :It sort the values by col1 in ascending order and col2 in descending order.
- df.groupby(col1): Returns a groupby object for the values from one column.
- df.groupby([col1,col2]) :Returns a groupby object for values from multiple columns.
- df.groupby(col1)[col2]) :Returns mean of the values in col2, grouped by the values in col1.
- df.pivot_table(index=col1,values=[col2,col3],aggfunc=mean) :It creates the pivot table that groups by col1 and calculate mean of col2 and col3.
- df.groupby(col1).agg(np.mean) :It calculates the average across all the columns for every unique col1 group.
- df.apply(np.mean) :Its task is to apply the function np.mean() across each column.
- nf.apply(np.max,axis=1) :Its task is to apply the function np.max() across each row.
Join/Combine
- df1.append(df2): Its task is to add the rows in df1 to the end of df2(columns should be identical).
- pd.concat([df1, df2], axis=1): Its task is to add the columns in df1 to the end of df2(rows should be identical).
- df1.join(df2,on=col1,how='inner'): SQL-style join the columns in df1 with the columns on df2 where the rows for col have identical values, 'how' can be of 'left', 'right', 'outer', 'inner'.
Statistics
The statistics functions can be applied to a Series, which are as follows:
Dataframe Cheat Sheet
- df.describe(): It returns the summary statistics for the numerical columns.
- df.mean() : It returns the mean of all the columns.
- df.corr(): It returns the correlation between the columns in the dataframe.
- df.count(): It returns the count of all the non-null values in each dataframe column.
- df.max(): It returns the highest value from each of the columns.
- df.min(): It returns the lowest value from each of the columns.
- df.median(): It returns the median from each of the columns.
- df.std(): It returns the standard deviation from each of the columns.

Some selected cheats for Data Analysis in Julia
Create DataFrames and DataArrays
df = DataFrame(A = 1:4, B = randn(4))
df = DataFrame(rand(20,5)) | 5 columns and 20 rows of random floats
@data(my_list) | Create a dataarray from an iterable my_list and accepts NA
df = DataFrame() ;df[:A] = 1:5 ;df[:B] = [“M”, “F”, “F”, “M”, “F”]
Importing Data
df = readtable(“dataset.csv”) | From a CSV file
df = readtable(“dataset.tsv”) | From a delimited text file (like TSV)
df = readtable(“dataset.txt”, separator = ‘t’) | With a tab delimiter
df = DataFrame(load(“data.xlsx”, “Sheet1”)) | From an Excel file using FileIO,ExcelFiles
df = readxl(DataFrame, “Filename.xlsx”, “Sheet1!A1:C4”) | From an Excel file using DataFrames,ExcelReaders
df = readxlsheet(DataFrame, “Filename.xlsx”, “Sheet1”) | From a whole sheet of Excel file using DataFrames,ExcelReaders
#Read from a SQL table/database
db = SQLite.connect(“file.sqlite”) # connection_object
df = SQLite.query(db,”SELECT * FROM tables”) | Read from a SQL table/database using SQLite and DataFrames
df = json2df(json_string) | Read from a JSON formatted string, URL or file using DataFramesIO
df_html = readtable(Requests.get_streaming(“http://example.com”)) | Parses a dataset from an html URL, string or file and extracts tables to a list of dataframes
DataFrame(dict) | From a dict, keys for columns names, values for data as lists
Exporting Data
writetable(“output.csv”, df) | Write to a CSV file
writetable(“output.dat”, df, separator = ‘,’, header = false) | Write to a CSV file
df2json(json) | Write to a file in JSON format using DataFramesIO and DataFrames
Viewing/Inspecting Data
head(df) | First 5 rows of the DataFrame
tail(df) | Last 5 rows of the DataFrame
head(df,n) | First n rows of the DataFrame
tail(df,n) | Last n rows of the DataFrame
size(df) | Number of rows and columns
length(df) | length of columns
nrow(df) | Number of rows
ncol(df) | Number of columns
showcols(df) | Show columns,missing,Datatype
describe(df) | Summary statistics for numerical columns
unique!(df) | View unique
values(df) or DataFrames.columns(df) | Return values or columns and their values.
Selection of Columns
df[:columnname] or df[“columnname”] | Returns column with label col as Series
df[1] | Select First column by number
df[[:col1, :col2]] or df[[Symbol(“col1”),Symbol(“col2”)]] | Returns columns as a new DataFrame
df[:, [:col1,:col2]] | Select specific columns of a dataframe and all rows of each column.
df[:, [1,3]] | Select specific columns of a dataframe
df[:, [Symbol(“col1”),Symbol(“col2”)]] | Select specific columns of a dataframe
s.iloc[0] | Selection by position
# Python => s.loc[‘index_one’] | Selection by index
df[1:3,[:col1,:col2]] | Selecting a subset of rows by index and an (ordered) subset of columns by name
#Python => df.iloc[0,:] | First row
df[1,:] | Select First row of all columns
# Python=> df.iloc[0,0] | First element of first column
df[1, 1] | First element of first column
# Python=> df.iloc[2:10] | Second row 2 to row 10 with all columns
df[2:10,:] | Second row 2 to row 10 with all columns
df[[1,2],:] Select row1 and row2 with all columns
# Python => df.loc[2]
df[2,:] | Select by index 2 all columns
Data Cleaning and Wrangling
names(df) | Names of columns
names!(df,[‘a’,’b’,’c’]) | Rename columns
rename!(df,:oldname,:newname) | Rename single column ,by modifying the original
showcols(df) | Shows the datatype,missing value of entire dataframe
isna.(s) | Checks for Missing NA in Arrray,Returns Boolean Arrray
isna.(df[:columname]) | Checks for null Values of a column, Returns Boolean Arrray
find(isna.(df[:,:columnname])) | Returns the individual rows of a column with missing values/na
.!isna.(df[:columnname]) | Returns a dataframe that contains no rows with missing values.
.!isna.() | Opposite of isna.() Solitaire free download mac.
dropna(df) | Drop all rows that contain null values
completecases!(df) | Drop all columns that contain null values like df.dropna(how=’all’)
df[isna.(df[:col1]),:col1] = x | Replace all null values with x
df[isna.(df[:col1]),:col1] = mean(df[:col1]) | Replace all null values with mean but mean must have no NA so use describe(df) and then its mean.
eltypes(df) | List Datatype of elements
convert(Array, df[:col1])| Convert the datatype of the series to float
indexmap(df[:A]) | Map the index
Filter, Sort, Groupby,Split Combine
df[df[:col1] .> 0.9] | Rows where the column col is greater than 0.9
df[(df[:col1] .> 0.2) & (df[:col1] .< 0.5)] | Rows where 0.5 > col > 0.2
sort!(df, rev = true) | Sort values by col1 in ascending order
sort!(df, cols = [:col1, :col2]) | Sort by col2 and col2
sort!(df,col1,rev=false) | Sort values by col1 in descending order
sort!(df, cols = [order(:col1, by = uppercase),order(:col2, rev = true)]) | Sort values by col1 byuppercase then col2 in descending order
sort!(df, cols = [order(:col1, rev = true),order(:col2, rev = false)]) | Sort values by col1 in ascending order then col2 in descending order Free movie download for macbook pro.
groupby(DataFrame,[:column]) | Returns a groupby object for values from one column
groupby(DataFrame,[:col1,:col2]) | Returns groupby object for values from multiple columns
aggregate(df,:col1,[size,length]) | Applying Aggregate of Functions to DataFrame with aggregate()
stack(df, [:col1, :col2; ]) | Reshape from wide to long format
melt(df, [:col1, :col2]) | Reshape from wide to long format ,prefers specification of the id columns
unstack(df, :id, :value) | Reshape from long to wide format
by(df, :columnname, df -> mean(df[:columnname2])) | Apply the function mean() across each column
colwise(mean, df) | Apply functions eg. mean to all columns
Join and Combine or Concat
# Append and Horizontal Concat
append!(df1,df2) | Add the rows of df1 to the end of df2 (columns should be identical)
hcat(df,df[:columnname]) | Add the columns in df1 to the end of df2 (rows should be identical)
join(df1,df2,on=:ID,kind=:left) | SQL-style join the columns in df1 with the columns on df2 where the rows for col have identical values. how can be one of ‘left’, ‘right’, ‘outer’, ‘inner’,’cross’
join(df1,df2,on=:ID,kind=:anti) | :anti => return rows that do not match with the keys
Statistics
describe(df) | Summary statistics for numerical columns
mean(df) | Returns the mean of all columns
mean(df[:col1]) | Returns the mean of columns 1
colwise(mean, df) | Apply functions mean to all columns
cor(df[:col1]) | Returns the correlation of a column in a DataFrame
counts(df[:col1]) | Returns the number of non-null values in a column of a DataFrame
maximum(df[:col1]) | Returns the highest value in column1
minimum(df[:col1]) | Returns the lowest value in column1
median(df[:col1]) | Returns the median of a column
mode(df[:col1]) | Returns the mode of a column
std(df[:col1]) | Returns the standard deviation of column1
# By Jesse JCharis
# Jesus Saves @ JCharisTech
# Inspired By DataQuest and J-Secur1ty
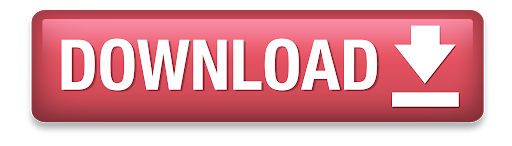